Generate synthetic datasets to accelerate model development in financial services.
For AI models to be effective at demonstrating human behavior in business scenarios, they need to be trained on large quantities of data that are representative of reality. The financial services industry generates large amounts of data which could be very beneficial, but such data is often not available for use. This poses a fundamental challenge for researchers and developers. Real data may be challenging to access along many dimensions including privacy, legal permissions, and technical aspects related to volume, representation, and meaning. The question is then, how to enable innovation and building of new products and services that depend on data.
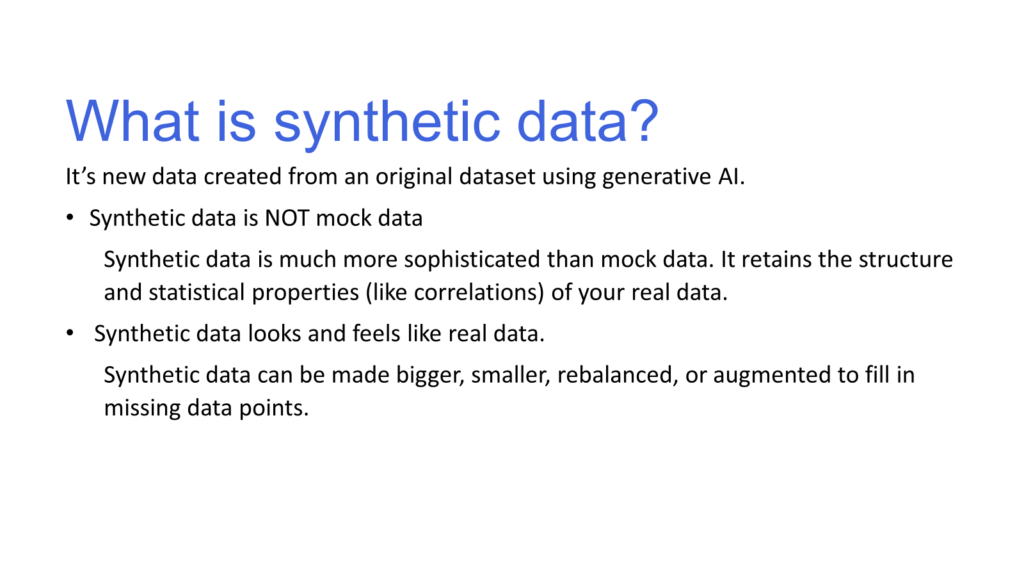
One answer is the use of synthetic data, which can share format, distributions and standardized content with the real data while not incurring the risks of using real data. Synthetic data potentially has the added benefit of representing exploratory scenarios beyond historical data to prepare AI algorithms and support decision making in novel situations. As such, synthetic data enables us to be more robust in our response to challenging situations.
Further, synthetic data can multiply examples that may be rare in the real data, in order to train machine learning algorithms more effectively. Ultimately, if a new idea shows promise on the synthetic data, we can consider advancing it for real deployment and use on the real data.
Through its research, the AI Research team at J.P. Morgan has identified several methods to create synthetic data and has learned that different methods may apply to different types of data. For example, they create realistic synthetic data by understanding the process that generates the real data, and then model the process itself to produce the synthetic data. The model can be declarative or captured in simulations. In addition, they can directly use the real data to train generative neural networks (GNNs), which have been successfully used to generate a variety of other synthetic data.
The synthesized new samples have properties of real data but cannot be mapped back to it. The new samples offer insight on data that otherwise may be left undiscovered.
One critical area is fraud detection model training where AI models are given examples of normal and fraudulent transactions in order to learn suspicious transaction patterns. Since the number of fraudulent cases is extremely small compared to non-fraudulent cases, modeling approaches struggle to effectively train models on fraudulent behaviors from the available data. However, synthetic data can be used to train a model on anomalous behavior. The process renders a greater percentage of transactions that do not fall in line with expected behavior, thus generating more synthetic samples of the fraud cases for improved model training.
Leveraging these techniques and others, the synthetic datasets that AI Research has developed include:
- Anti-money laundering (AML) behaviors
- Customer journey events
- Markets execution data
- Payments data for fraud detection
Manuela Veloso, Head of AI Research at the firm, reflected on synthetic data capabilities the team has enabled in retail banking. “Synthetic data generation allows us to think, for example, about the full lifecycle of a customer’s journey that opens an account and asks for a loan. We’re not simply examining the data to see what people do, but we’re also able to analyze their interaction with the firm and essentially simulate the entire process.”
Key Benefits
Data Richness and Diversity: Synthetic data generation provide Banks with access to a broad spectrum of data, encompassing scenarios that had not yet occurred but were plausible. This diversity improves the predictive capabilities of its risk management models.
Risk Model Accuracy: Enhanced by synthetic data, Banks\' models could detect and predict fraudulent activities and credit risks with higher precision, reducing the incidence of false positives and negatives.
Regulatory Compliance: Using synthetic data helps Banks navigate the regulatory landscape by ensuring that customer privacy was upheld, reducing the risk of data breaches and non-compliance penalties.
Cost Efficiency: Generating synthetic data proved to be cost-effective, eliminating the need for expensive data acquisition from third parties and reducing the reliance on manual data anonymization processes.
Conclusion
Banks\'s strategic use of synthetic data generation for risk management exemplifies the transformative potential of Generative AI in the banking sector. By enriching its data environment, Banks can enhance the accuracy and efficiency of its risk management practices, ensuring higher standards of customer privacy and regulatory compliance.
Fintech vendors in this space